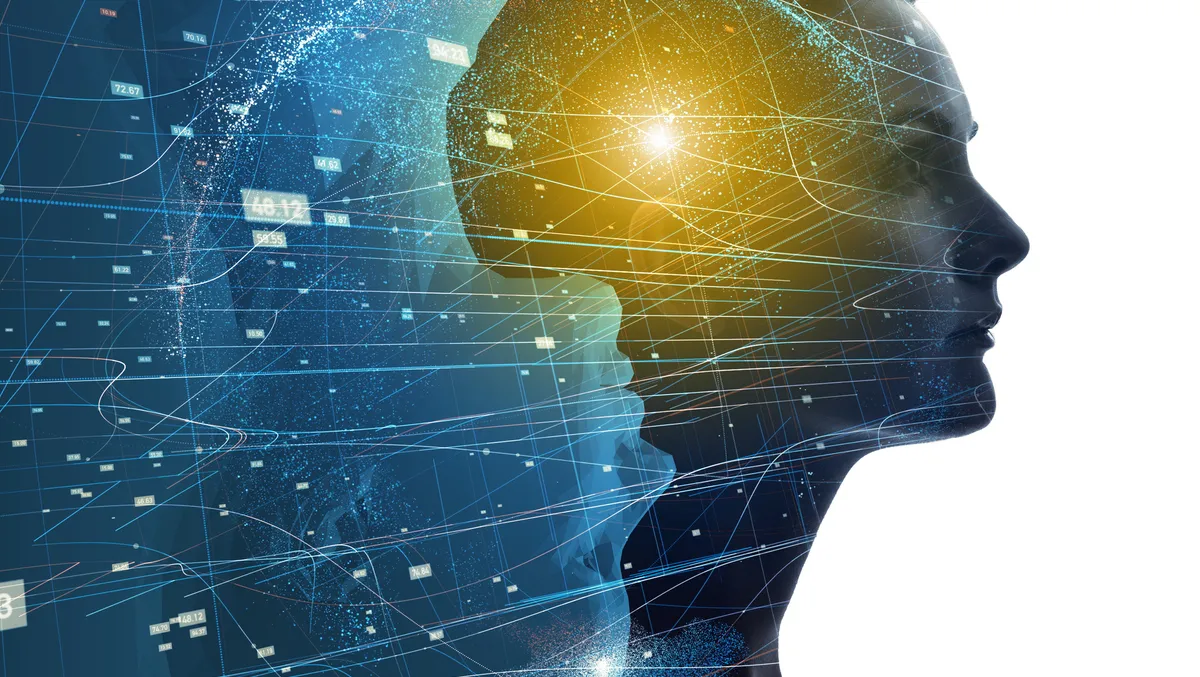
Don't allow bad data to ruin your digital twin plans
Old machinery retrofitted with sensors, multiple IoT devices from varying manufacturers, event streaming and the sheer volume of collected data are creating a perfect storm of problems for any business looking to use digital twin technology to simulate and monitor real-world activities. The problem is that so much of this data is either inconsistent, inaccurate, or incomplete that it cannot be trusted as a source for creating simulations. It's a risk and one that needs addressing urgently to avoid wasted budgets and poor decisions.
For digital twins, poor data quality is the Achilles heel. IDC predicts in its Global DataSphere that the volume of data generated annually will more than double from 2021 to 2026, which will only make the situation worse if it is not addressed, as data quality tends to decrease with increased volumes.
For the burgeoning digital twin market, this could be disastrous. Digital twins are being widely adopted across industries and rely on quality data to create accurate simulations of real-world scenarios. In manufacturing, construction, city planning, environmental monitoring, transportation and healthcare, digital twins have already found a home. And they are only going to grow in influence.
It's only going to get bigger, more widespread and more mission-critical to so many industries and organisations, so the ability to replicate, measure, monitor, predict, test and simulate in real-time means that accurate data is essential.
It's easy to see why. Imagine the scenario where the underlying machine learning and AI algorithms are designed using poor-quality data. Imagine this also running within digital twins built using the same poor-quality data. It exacerbates the problem and will lead to inaccurate anomaly alerts and predictions that cannot be trusted. In short, a waste of time and money, with what is an excellent technology for actually saving time and money.
We know this because we have seen it in action. Spanish manufacturer CAF has built a digital twin to help its railway operation and maintenance customers achieve operational excellence through agile decision-making. The digital twin enables CAF to automate activities without the need to send anyone to check fleets or a specific train. It can feed its engineering teams with real data to better operate and maintain fleets.
So, what do organisations need to do to create a better, more accurate, less risky twin? Quite simply, the systems need to be built on good data and trained to continuously monitor that data to maintain a level of quality. This is a central characteristic of modern data architectures, and many platforms can increase data quality, creating trust in data while also preventing inaccurate data.
Risk factor. A five-point plan
It's also about breaking down silos to ensure a broad sweep of contextual data sources, integrating that data and driving better outcomes for decision-making. In a data architecture, increasing data quality is a five-step, iterative process:
- Integrate data sources from various systems with data virtualisation and real-time sources
- Profile data to discover and analyse where source data needs to be fixed or improved
- Proceed with manual data remediation to fix issues from previous steps
- Automate data cleansing and deduplication based on models and rules
- Monitor data over time and provide KPIs to understand data trends
It's also possible to create a data quality 'firewall'. The last thing any organisation wants is to allow poor data back into the system after it has been cleansed. This 'firewall' can provide real-time error detection and correction to protect the digital twin, ensuring that any data ingested reaches defined quality levels. Of course, data can be cleansed and corrected, and a good platform can do this in real-time from any type of data source.
You can also do this retrospectively, cleaning data and potentially blocking consistently poor sources of data. This is an important feature, given the prevalence of legacy machines and technologies. For organisations to benefit from digital twins now, they do not want to rip and replace machines and systems but try and work with what they have.
However, given the increasing importance of the decisions that organisations want to make based on digital twin simulations, it is imperative that data quality is at the forefront of their thinking. Decisions may impact a patient's life, slow down a manufacturing process, delay trains or send field service engineers on unnecessary maintenance jobs. It should be easy to see why data quality is a must, especially when AI/ML models are trained and used for integral decision-making. In truth, anything less is asking for trouble.