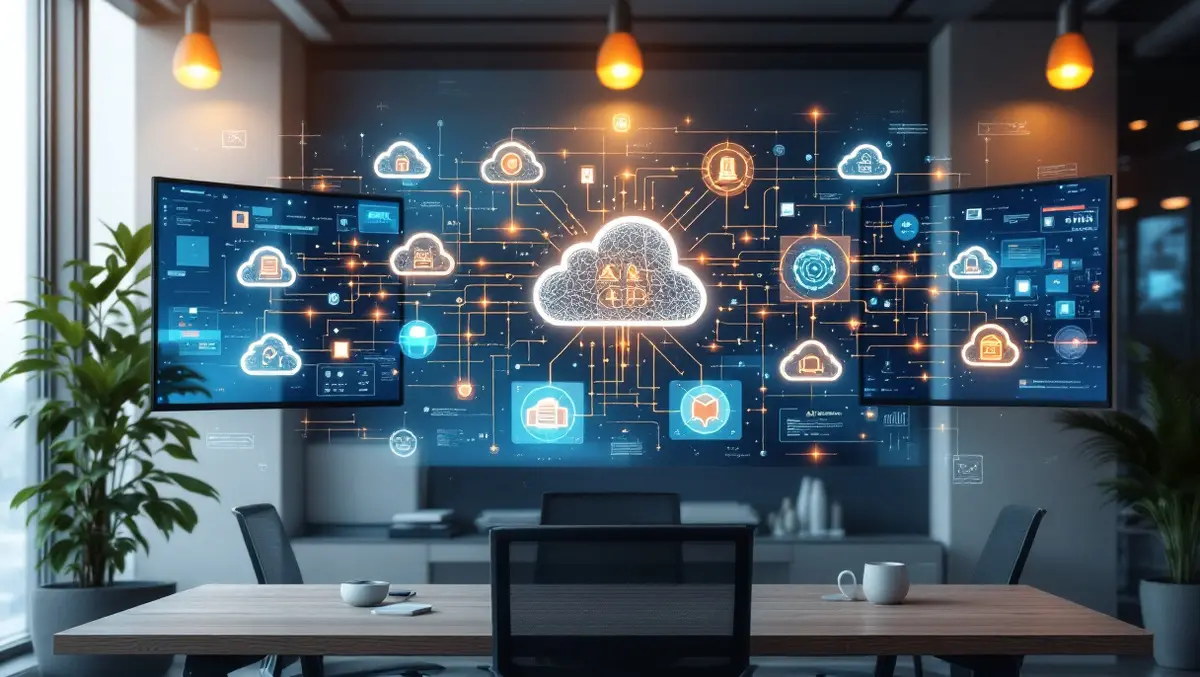
Starburst rolls out AI features for unified data access
Starburst has announced a suite of new features across its Starburst Enterprise Platform and Starburst Galaxy aimed at unifying access to data for enterprise AI projects.
The company's latest updates are designed to address challenges faced by organisations whose data is fragmented across on-premises, multi-cloud, and hybrid environments, making it harder to implement AI initiatives efficiently and securely. These capabilities are expected to help enterprises unlock modern data lakehouse architecture for AI applications, apps, and analytics.
AI projects typically require integration across disparate systems, which can involve complicated pipelines and a lack of consistent governance. According to Starburst, this can slow experimentation, raise risks, and delay results due to difficulties accessing the correct data, involving stakeholders, and enforcing compliance policies.
Justin Borgman, CEO and Co-Founder of Starburst, commented, "AI is raising the bar for enterprise data platforms, but most architectures aren't ready. At the end of the day, your AI is only as powerful as the data it can access. Starburst is removing the friction between data and AI by bringing distributed, hybrid data lakeside, enabling enterprise data teams to rapidly build AI, apps, agents, and analytics on a single, governed foundation."
Among the significant updates is the introduction of Starburst AI Agent, a conversational natural language interface allowing data analysts and AI agents to generate insights directly from governed data within a secure Starburst environment. Starburst also rolled out AI Workflows, which streamline the process from experimentation to production for AI use cases by connecting vector-native search, context from metadata, and governance in an open data lakehouse architecture.
Rob Strechay, Managing Director & Principal Analyst at theCUBE Research, said, "Starburst uniquely helps enterprises speed up AI adoption, reduce costs, and realise value faster by enabling access to all their data, no matter where it lives, across clouds, on-premises, or hybrid environments. The best part? Because data has gravity, they don't need to move or migrate it to build, train, or tune their AI models."
Matt Fuller, VP of AI/ML Products at Starburst, stated, "We're turning the data lakehouse into an enterprise-grade platform for AI agents and applications - designed from the ground up to support air-gapped environments without compromising on flexibility. Whether deployed in a secure on-premise environment or cloud-enabled ecosystem, Starburst delivers federated, governed access, real-time context, and high performance query processing. We're not just accelerating AI innovation; we're operationalising it, securely and at scale."
Additional functionality now available or in preview includes Galaxy's AI-powered Auto-Tagging, which uses large language models to detect sensitive data such as personally identifiable information (PII) at the column level. Human-in-the-loop review and support for custom classifiers are intended to strengthen governance and enable scalable Attribute-Based Access Control (ABAC) policies.
Starburst has also released Starburst Data Catalogue, a new enterprise-grade metastore intended to replace Hive Metastore, with support for Apache Iceberg and future multi-engine integrations, aiming to reduce metadata sprawl and improve governance while avoiding vendor lock-in.
Enhancements in data management show up in Galaxy's fully managed Iceberg Pipelines. This feature offers automated maintenance with options for streaming ingest from Kafka or batch ingestion from S3, and capabilities such as compaction and orphan file removal to ensure high performance and cost-efficiency for Iceberg tables. According to Starburst, this is designed to require no manual tuning from users.
New tools like a Starburst-native ODBC driver and advanced features for Starburst Enterprise target query performance and operational efficiency, including scheduled materialised view refreshes and automated Iceberg table maintenance. Starburst is also offering automatic query routing in Galaxy, using load or user role to determine the appropriate cluster for each query, with the aim of improving performance and cost control.
Starburst: Data-to-AI Readiness Blueprint is introducing a new service offering to help customers assess and improve their data infrastructure for future AI workloads. This engagement includes evaluating current architecture, streamlining integration across complex environments, and providing a tailored roadmap for data infrastructure for scalable and secure AI outcomes.
Pedro Pereira, Staff Data Engineer at Going, commented, "Since choosing Starburst Galaxy as the core of our Lakehouse architecture, we've been using the built-in Streaming Ingest capability to land large volumes of flight and user data...At the conclusion of our testing, we were left thoroughly impressed with the functionality, performance, and ease of use. Similarly, Galaxy Orphan File Removal exceeded our expectations by saving us an estimated USD $1,000/month in S3 storage costs..."
Ricardo Cardante, Staff Engineer at TalkDesk, stated, "Before Starburst, maintaining our Iceberg tables was a manual, error-prone process that only covered a fraction of our data. With Automated Table Maintenance, we applied compaction and cleanup across the board, going from 16% table maintenance coverage to 100%. This enhancement led to a 66% reduction in S3 storage costs for our data platform buckets and contributed to an overall 20% decrease in S3 storage expenses across the company. It's a game changer for scaling Iceberg performance with minimal overhead."
George Karapalidis, Director of Data at Checkatrade, said, "User Role-Based Routing in Galaxy made it simple to direct queries to the right cluster based on team roles. It's intuitive, seamlessly integrated into the Galaxy UX, and helps us optimise for both performance and cost without adding operational overhead."